Eyes on the Prize: Challenges Using AI in Ophthalmology
Eyes on the Prize: Challenges Using AI in Ophthalmology
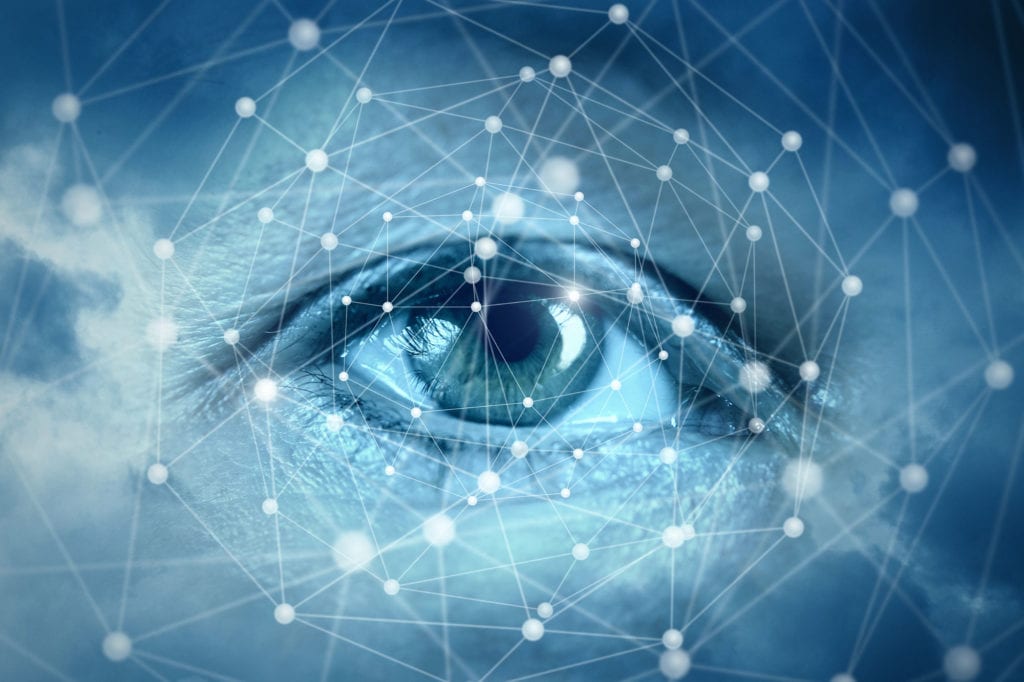
The use of artificial Intelligence (AI) in global healthcare is expected to expand at a compound annual growth rate of 38.4% from 2022 to 2030. While AI is driving compelling innovation across healthcare, including the ophthalmology space, it brings a new set of challenges. In order for AI to fulfill its promise of increasing efficiency and lowering costs for ophthalmic research and trials, there are several challenges to address first.
Issues & Limitations of AI in Ophthalmology
Error handling for real-world applications: The technical immaturity of error handling of AI processes is a concern when it comes to clinical validation and regulatory approval of the algorithms. Before contemplating using AI in a clinical setting, many new logistical and operating processes must be defined so that errors can be quickly identified and resolved.
Massive data storage: AI is capable of analyzing large amounts of data and generates better results as more data is processed and fed back through into algorithm training. However, the question of who should be legally and financially responsible for the storage and security of sensitive patient data remains an open question.
Regulatory concerns: The FDA is the primary gatekeeper for clinical devices and software as a medical device (SaMD). Its primary role is to act in the patient’s interest to ensure the safe and effective deployment of not only devices in ophthalmology, but also therapeutics, surgery, and treatment. Keeping apace of AI-based SaMDs from algorithms that “denoise” images to those that predict patient outcomes is a tall order, and there’s no doubt approvals will take longer as a result.
Legal implications of misdiagnosis: The regulations surrounding who holds responsibility in case of a misdiagnosis by AI is still a major question mark. Since most current AI algorithms diagnose only one disease, there is room for error by omission, and a correct AI diagnosis is by no means a comprehensive clinical workup.
Ethnic and regional diversity: Certain eye diseases, progressions, and treatments can have ethnic and regional variations. The movement to build more ethnically/regionally diverse AI is still very much in its early stages. Many concerns have been raised about requiring data from all over the world to build a broad enough population set to minimize bias and maximize global utility. The chronic issues in impoverished regions of limited health care and lack of infrastructure will also impact the ability for AI to be adopted across the globe.
Interoperability: In order to make AI in ophthalmology more practical there is a need to use data from any of the multitude of systems and software formats so that the images can be read and analyzed from any device. In radiology, this is made possible via DICOM. Ophthalmology is far behind here with most devices still outputting data in vendor-specific, non-DICOM format.
We are losing many opportunities to utilize ophthalmic image data for developing AI systems because those data are locked in proprietary standards and inaccessible to researchers and clinicians.
Michael F. Chiang, Director, National Eye Institute, National Institutes of Health, Bethesda, Maryland, USA
Trial recruitment: Identifying and selecting clinical trial patients can certainly be made easier with AI. However, there are still limitations within machine learning that need to be addressed when it comes to determining the eligibility of these patients, as the algorithms are designed to identify only one eye disease at a time.
Human Errors: Implementing AI requires an initial training data set, and external validation. Data scientists must label the data in the training process of the algorithm, which adds a risk of human error. This is also a very time-consuming project and requires the development of and adherence to standards to ensure the accuracy of AI algorithms and systems as they are rolled out around the world. The garbage-in, garbage-out analogy is particularly apt here.
The Future of AI in Ophthalmology
While there are still challenges that need to be addressed, the potential for AI in ophthalmology is undeniable. Keeping the tremendous upside of AI in mind should encourage the ophthalmic research community to take on these challenges and iterate toward some workable solutions. As ophthalmic technology further evolves, it will bring us closer to a future where AI can assist in better solutions for some of our most pervasive eye diseases.
This is the second blog in our 3-part series. Read part 1: Eyes on the Prize: The Case for AI
To learn how Orion enables AI-backed analysis, provides interoperability in ophthalmic image analysis, and reduces read times with automated measurements, visit voxeleron.com or get in touch to request a demo.
Sources:
Cybersight Lecture: Artificial Intelligence in Ophthalmology
The Impact of Artificial Intelligence in Ophthalmology
AI in Ophthalmology: Challenges and Way Ahead