Introducing RPE-Baseline
In SD-OCT volumetric imaging you cannot always see Bruch’s membrane, the innermost layer of the choroid, lying at the base of the retinal layers. It is an important structural landmark for a variety of reasons and one that is important, therefore, to delineate in automated analysis. If it could always be reliably seen, it would be best to just explicitly segment it. Indeed, this is the approach we take with the Spectralis data where the data is highly averaged and the membrane is usually visible as a result. More commonly, the data from instruments such as that from Zeiss, Topcon and Optovue is slightly noisier due to the lack of averaging and the membrane cannot be resolved. On the other hand, the data is more isotropically sampled and consequently more volumetric. Such rich data cubes do permit more inherently three-dimensional segmentation techniques, which is certainly advantageous in terms of overall accuracy. Some of these techniques are shape-based methods, which can typically be successively honed becoming more descriptive through the mining of large data populations. Taking advantage of these factors, we have released an automated delineation of the RPE-baseline, pertaining to Bruch’s membrane, for Cirrus data in our OrionTM Software.
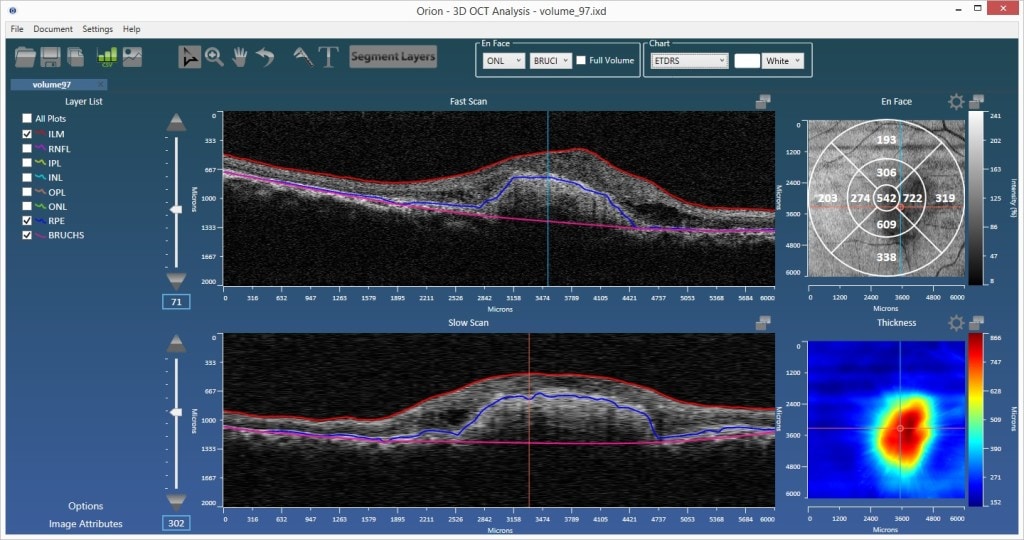
Existing Methods and the Importance of an RPE-Baseline
In certain pathologies, Bruch’s membrane can remain relatively constant, while the RPE above it can change dramatically. Measuring RPE elevations relative to the baseline of Bruch’s has utility in the management of non-exudative and exudative AMD, but only if it is measured well.
The literature is limited in this space as the gold-standard for drusen measurement is using color fundus photographs, and, as a result. the majority of automated methods have been based on such 2d imagery. In the advent of OCT’s volumetric imaging, the algorithms had to change, but in general, the methods have involved a simple fitting of of a surface to the data. Though elegantly simple, the methods have, unfortunately, their own drawbacks. Firstly, when fitting to a segmentation of the RPE exhibiting pathology, it omits no characteristic shape, meaning the quality of fit is not guaranteed. Secondly, it is difficult to appropriately constrain to fit only the healthy RPE locations. Consider, for example, the case where drusen are prevalent. Your fit will likely dissect the druse somewhere above their base as the overall fit is pulled upward. Instead, a baseline surface needs to be more explicitly define based on 1) knowledge of the non-pathological areas and 2) a higher level understanding of the characteristics shapes the human retina can assume. The RPE-baseline method we’re introducing into our OrionTM software, does both of these, and thereby offers significant advantages over existing, fitting techniques.
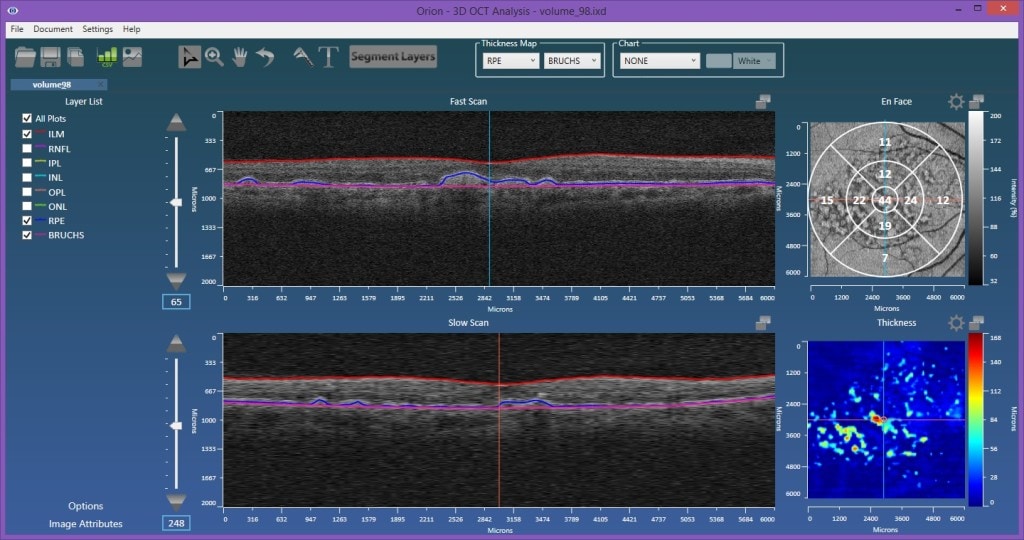
The Literature
The most telling contribution in this area is from Bascon Palmer [1], a center known for developing algorithms and having outstanding clinical support. Interestingly, this algorithm was commercialized into the Cirrus OCT family of devices. The resulting RPE elevation map is shown in their literature here, with the interesting words of caution:
“The minimum RPE elevation that the software will include in the quantitative result is 19.5 µm.”
We’ve no way of knowing what the method is, but if you have to filter the result to axially ignore in this case 10 pixels of elevation, then it is most likely that the surface can be described mathematically as a smooth surface, and one that also gets pulled upward. The danger here is again that, where drusen or edema and so on proliferate, your estimate of volume due to pathological elevations of the RPE is significantly underestimated as a result of erroneously raising the baseline.
Chiu et al. [2] have a more explicit mechanism for their RPE baseline as they employ a segmentation technique popular in that lab, graph traversal. Such methods are locally optimal based on parameters of the algorithm and the cost images. In general they are too explicit, and a method working in specific drusen cases, and not able to generalize to other topographies, that might include PEDs, for example. Interestingly, an earlier version from the same lab did use fourth order polynomial fitting [3], in this case for much larger looking drusen. Scale is certainly something that might warrant use of different methods.
More recently, Chen et al. [4] look to characterize the RPE baseline, but again with fitting. The RPE itself is segmented using thresholding and iterative outlier rejection, and drusen refinement is based on the en face view only. The fitted surface is polynomial and suffers issues at the borders of the image, another caveat to the fitting approach.
More on the Importance of RPE Baseline
The ability to quantify various stages of AMD, including both drusen and choroidal neovascularization is extremely important in following disease progression. Equally, for management treatment response in clinical trials, such biomarkers can be crucial.
Image segmentation is critical in quantitative analysis and provides such endpoints. But layer segmentation is also becoming a critical component as an enabling technology in other errors. OCT angiography is completely reliant on layer segmentation to identify areas of perfusion/non-perfusion. The plexuses that are analyzed are defined by a top layer and a bottom layer, provided automatically through segmentation. Choroidal imaging in OCT angiography, in particular imaging of the choriocapillaris, is of tremendous interest among the ophthalmic community. Having a robust baseline at the interface of the retina and the choroid facilitates this, even in the presence of advanced ocular pathologies.
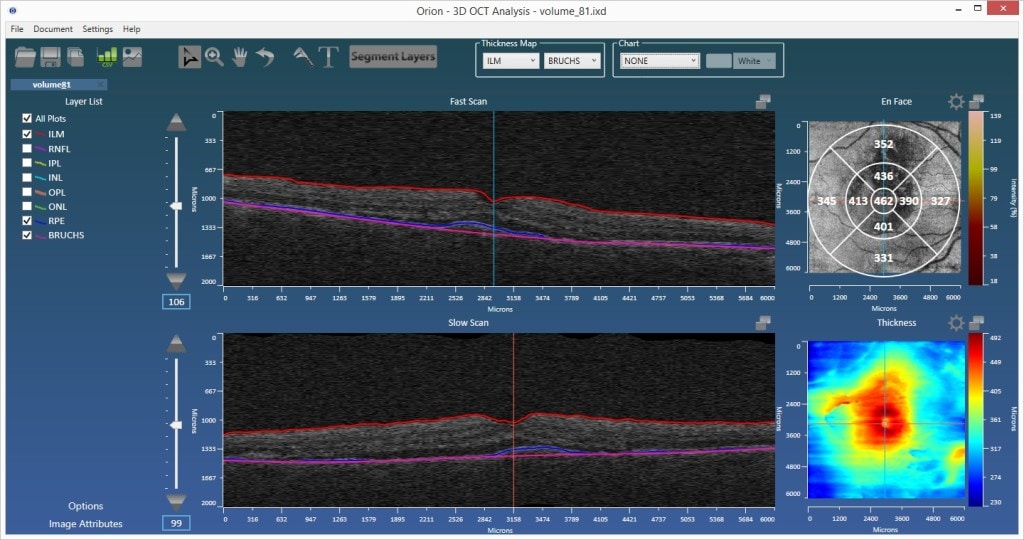