Voxeleron at ARVO 2019
Voxeleron at ARVO 2019
Orion™ Updates
In active use worldwide, Orion has now processed over 48,000 OCT volumes. This is robust, mature software and as a reminder, the only commercial-grade alternative to the slow, stale algorithms that ship with the instruments. While our OCT analysis software has undergone a number of iterative changes, the main updates readied for this year’s ARVO are:
- OCT Angiography Analytics – we now have more endpoints than you can shake a stick at, including:
- Vascular density – based on automated thresholding as well as fractal analysis.
- Foveal avascular zones – again fully-automated, defined for all plexuses and editable.
- Visualizations – we have two different approaches: one to support capillary visualization; and also a deep learning-based segmentation that extracts the main vascular trunk.
- Choroid Segmentation – this is work that we originally submitted to the Imaging in the Eye meeting that precedes ARVO. Based on the exciting results, we thought it best to integrate the algorithm into Orion and make it available to those users who had been asking for just this functionality. It’s based on SD-OCT data, which is all the more challenging as the choroid / sclera interface can be pretty hard to see. Indeed, that motivated the method, a deep learning approach using what is being called a V-Net – a U-Net for volumetric data. It means that, for the first time in Orion, we utilize GPUs to perform the segmentation. A further word on this topic is below.
- Export to DICOM – we think that this is a big deal in terms of workflow for CRO’s and reading centers. It basically makes existing infrastructure – databases, storage, applications – compatible with all OCT devices. Orion then acts as the unifying analysis, visualization, and editing tool, and exports the image data and results to DICOM – a universal format which can then be transferred back into existing analysis and reporting pipelines. Not only does this dramatically speed up workflow, it will hasten clinical trial recruitment as the restriction to a single device type is removed. It’s a piece of the clinical trial puzzle that no other software can address. And that’s not even considering the fact that Orion is the most advanced OCT analysis software available….
- Minor updates:
- More image data support, including some of the newer Optovue scan patterns as well as Bioptigen data (both using file extension .OCT).
- In addition to the standard ETDRS and Quadrant grids, our GCIPL analysis now reports the annulus described in an IOVS publication.
- Minor improvements, modifications to the formatting of the reporting mechanisms (CSV and XLS), and some speedups.
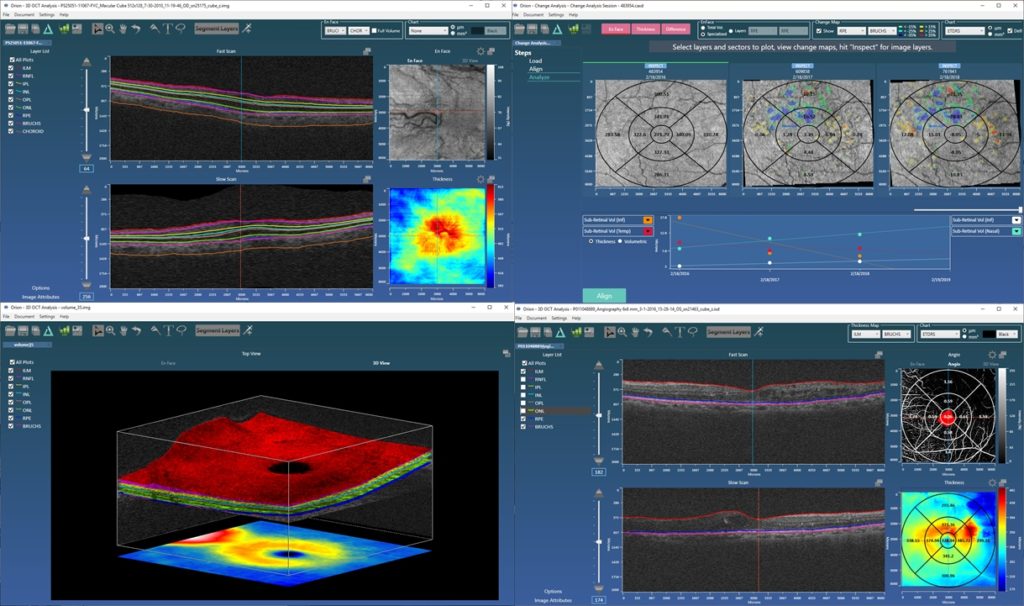
Deep Learning
We natively support deep learning algorithms in Orion. This is not actually new as we first demonstrated our fast review functionality – DeepSee – at ARVO in 2017. There have been a lot of developments in this area, however, so we have moved to the latest CUDA libraries to support our current releases. This does mean that, to take advantage of the GPU-based processing for these specific algorithms, your PC will need a suitable NVIDIA graphics card. Customers without a GPU will still have access, of course, to Orion’s standard suite of lighting-fast segmentation and analysis algorithms, just not the deep learning-based stuff.
Orion in Press
- “Visual Function and Disability Are Associated With Focal Thickness Reduction of the Ganglion Cell-Inner Plexiform Layer in Patients With Multiple Sclerosis”, Ce Shi; Hong Jiang; Giovana Rosa Gameiro; Huiling Hu; Jeffrey Hernandez; Silvia Delgado; Jianhua Wang. Investigative Ophthalmology & Visual Science March 2019, Vol.60, 1213-1223.
- “Age-related Alterations in Retinal Tissue Perfusion and Volumetric Vessel Density”, Ying Lin, Hong Jiang, Yi Liu, Giovana Rosa Gameiro, Giovanni Gregori, Chuanhui Dong, Tatjana Rundek, and Jianhua Wang. Invest Ophthalmol Vis Sci. February 2019, Vol.60, 685-693.
- “Deep Learning for Prediction of AMD Progression: A Pilot Study”, Daniel B. Russakoff, Ali Lamin, Jonathan D. Oakley, Adam M. Dubis, and Sobha Sivaprasad. Invest Ophthalmol Vis Sci. February 2019, Vol.60, 712-722.
- “Changes in volume of various retinal layers over time in early and intermediate age-related macular degeneration”, Ali Lamin, Jonathan D. Oakley, Adam M. Dubis, Daniel B. Russakoff & Sobha Sivaprasad. Eye (2018)
- “Visualization of Focal Thinning of the Ganglion Cell–Inner Plexiform Layer in Patients with Mild Cognitive Impairment and Alzheimer’s Disease”, Y Shao, H Jiang, Y Wei et al. Journal of Alzheimer’s Disease, vol. 64, no. 4, pp. 1261-1273, 2018.
Deep Learning for AMD Prognostics
We were particularly pleased to see our collaborative work with Moorfields/UCL published in IOVS at the beginning of this year. An earlier paper had used Orion to look at structural changes in the inner and outer retinal layers over time in early and intermediate age-related macular degeneration (AMD). We extended that work to look at how, at baseline, the OCT data could be prognostic of conversion from early to advanced AMD. For this, we again used deep learning to build an inference network that, based on the OCT data alone, scored the likelihood of conversion. A cross-sectional analysis of the data at baseline offers no known biomarkers that are predictive of future change, so the use case is compelling. The work looks at various network architectures, but critical to the excellent predictive performance is the pre-processing of the OCT data that uses Orion’s segmentation. This crops the data from the inner limiting membrane (ILM) to an offset to Bruch’s allowing the network to concentrate on the data relevant to the question being asked; that is, OCT data in the vitreous and posterior to the choroid is ignored. The cropping also has the effect of normalizing the data allowing the study to use data from different devices.
Such network architectures are particularly good at finding signal in diffuse patterns of data – tasks human readers find particularly difficult – and as our occlusion analysis demonstrated, the networks found relevant information from the retinal pigment epithelial layer (RPE) down. This makes sense as it suggests RPE integrity, Bruch’s and the choriocapillaris are involved, which confirms clinical understanding. And furthermore, it is exactly where researchers using OCT-A are looking for sub-clinical choroidal neovascularization (CNV) assessment.
Next Steps
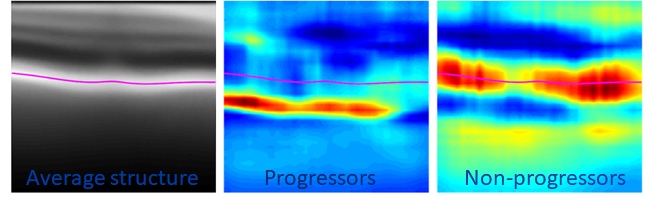
ARVO 2019 Posters and Sessions
- “Automated choroid segmentation of SD-OCT volumes using deep learning.” Oakley, Jonathan D. and Russakoff, Daniel B.
- ARVO Imaging in the Eye Meeting, Saturday April 27th, 10:00 -10:30 AM, 12:15 – 1:30 PM, 3:15 – 3:45 PM. Session Number: 007; Session Title: Artificial Intelligence. Presentation #: PB0109.
- “Diagnostic Assessment of RNFL Segmentation using a Hybrid Deep Learning Approach.” Oakley, Jonathan D., Mannil, Suria S.; Russakoff, Daniel; Chang, Robert.
- ARVO, May 1, from 3:00 PM to 4:45 PM. Presentation / Posterboard #: 5599 / B0490. Session Number: 495; Session Title: Imaging II.
- “A 3D Deep Learning System for Detecting Referrable Glaucoma Using Full OCT Macular Cube Scans.” Russakoff, Daniel; Mannil, Suria S.; Oakley, Jonathan D.; Chang, Robert.
- ARVO, April 29, 2019 from 8:15 AM to 10:00 AM. Presentation / Posterboard #: 1474 / A0158. Session Number: 218; Session Title: Machine Learning I.
- “Novel deep learning based algorithm for Macula and Optic Nerve Head segmentation versus Cirrus Optical Coherence Tomography in identifying glaucoma.” Sudhakaran Mannil, Suria; Oakley, Jonathan D.; Russakoff, Daniel B.; Chang, Robert.
- ARVO, May 1, 2019 from 3:00 PM to 4:45 PM. Presentation / Posterboard #: 5558 / B0449. Session Number: 494; Session Title: Imaging I.
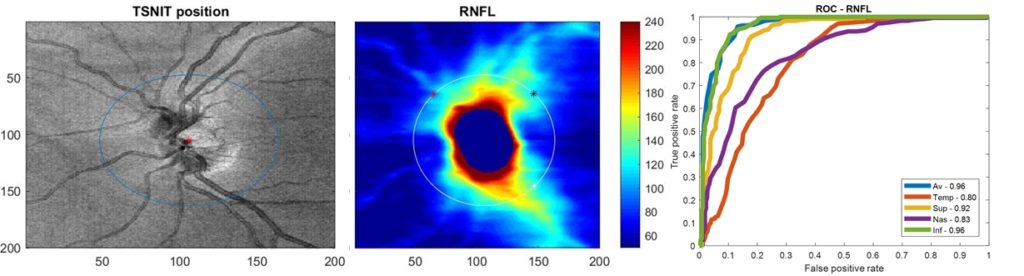
Voxeleron Bits and Bobs
- Voxeleron and Social Media – We were excited to be invited to record a podcast as part of the Dell Luminaries series. We’re very happy to use their equipment for our deep learning tasks. You can hear the podcast at this link.
- AAO: if you will miss ARVO, do keep in touch as we’ll be at AAO this year. It’s in San Francisco, so we’ve no excuses for missing this one!
- We tend to end this newsletter with a roadmap update, and this’ll be no different. The question is, what next for Orion? Is it:
- More deep learning-based analyses?
- Optic nerve head analysis, leveraging the recent work done for ARVO (see posters above).
- Something completely different?
And Finally
We hope that you have found these updates to be of interest. It’s worth mentioning here that most of these are driven by your input as we work in as collaborative a way as possible. As this is research software, we’re able to respond very quickly to user requests as the software is only there to support your research. So please, if you can’t make ARVO do reach out via email. We would be delighted to hear from you.
With best regards,
The Voxeleron team.